ChBE, ECE early career researchers will use the five-year grants to speed computer hardware design, study movement of nanoparticles, and improve understanding of graph-structured data.
(text and background only visible when logged in)
Georgia Tech engineering faculty members Callie Hao, Vida Jamali, and Pan Li have received sought-after funding from the National Science Foundation (NSF) to help them establish leadership in their fields.
The agency’s Faculty Early Career Development Awards are highly competitive five-year grants designed to help promising scholars build the foundation for their research. Known as CAREER awards, the grants are for those who have the potential to serve as role models in research and education.
Meet the researchers and explore how they’ll use their grants for difference-making projects:
(text and background only visible when logged in)
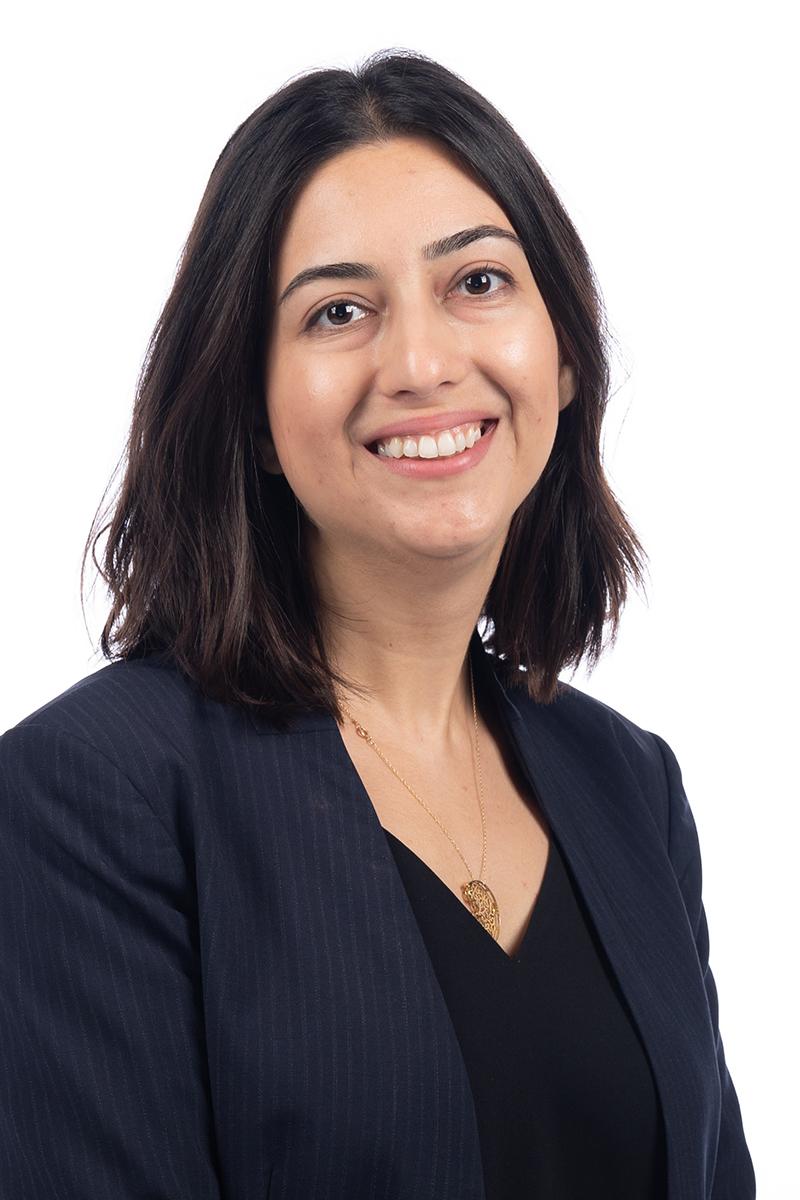
Vida Jamali
Assistant Professor and Daniel B. Mowrey Faculty Fellow
School of Chemical and Biomolecular Engineering
Jamali will use new imaging technology and machine learning to understand the movement of single nanoparticles and their interactions with their nearby environments. Nanoparticles are smaller than a strand of human DNA.
How nanoparticles move and interact with surfaces and other environments is important in nanomedicine, environmental remediation, sensing applications, and more. Jamali will be able to visualize and characterize the movement of these particles by the millisecond.
"By combining the emerging in situ liquid phase transmission electron microscopy, nanoscience, machine learning, and stochastic thermodynamics, we will develop a new single particle tracking technique that will enable probing of the dynamics of single particles’ motion and interaction at a length scale inaccessible before," Jamali said.
Callie Hao
Sutterfield Family Early Career Professor and Assistant Professor
School of Electrical and Computer Engineering
Hao is working to speed the design of new computer hardware architectures using what are known as High-Level Synthesis (HLS) tools. HLS digitally creates hardware designs based on algorithmic specifications. This approach to designing digital circuits and computer architectures can save time and effort compared to the conventional approach.
HLS tools have limitations, however. They are good at synthesizing a specific algorithm into hardware but are less effective when creating general domain-specific architecture designs — specialized computer hardware designed to work in a particular domain, such as image processing. HLS tools also struggle to improve the underlying architecture as the computing workload evolves.
Hao will work to unlock more widespread application of HLS by developing a next-generation tool. Called ArchHLS, the tool will address the shortcomings of existing HLS tools and be publicly available.
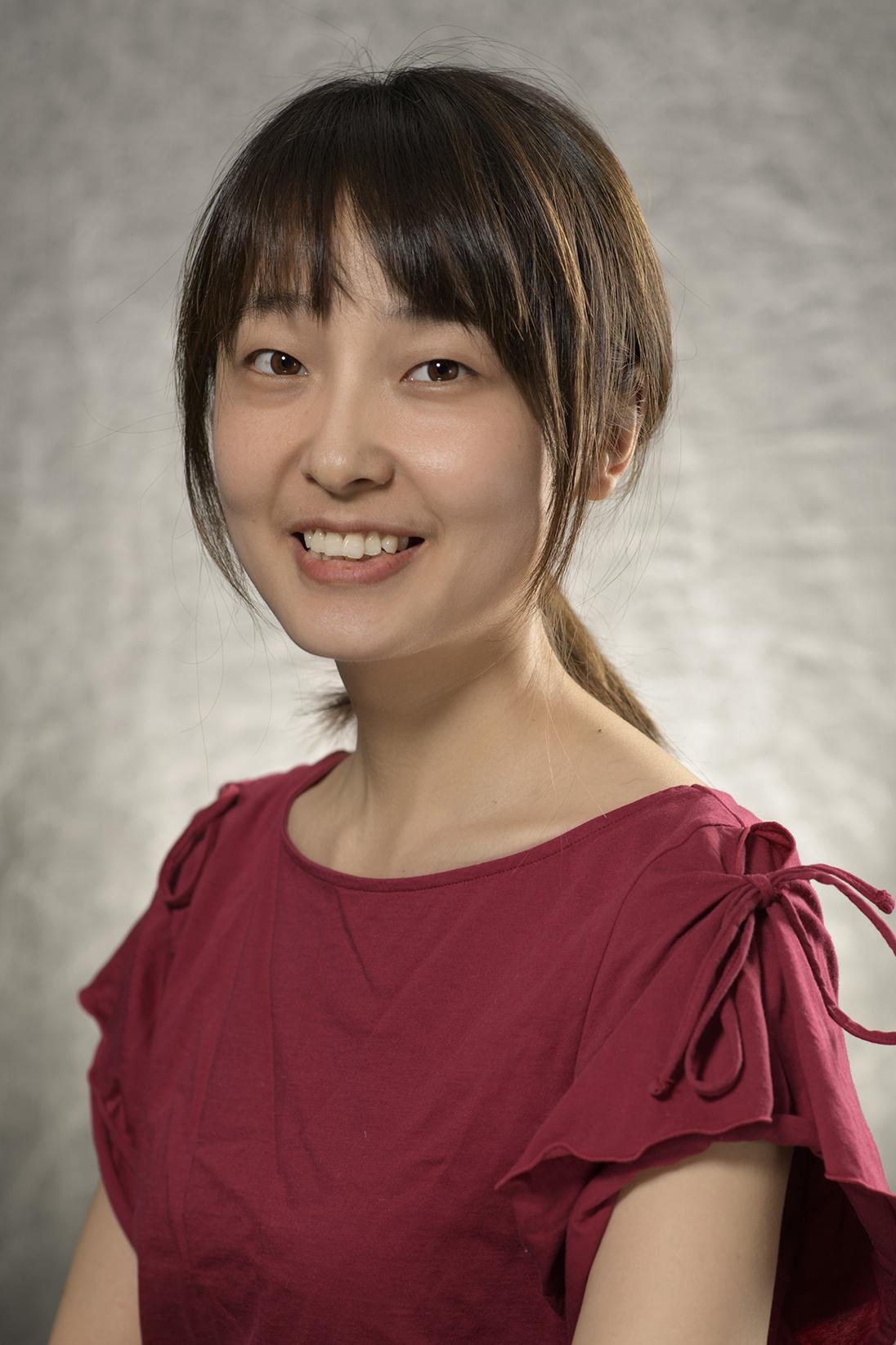
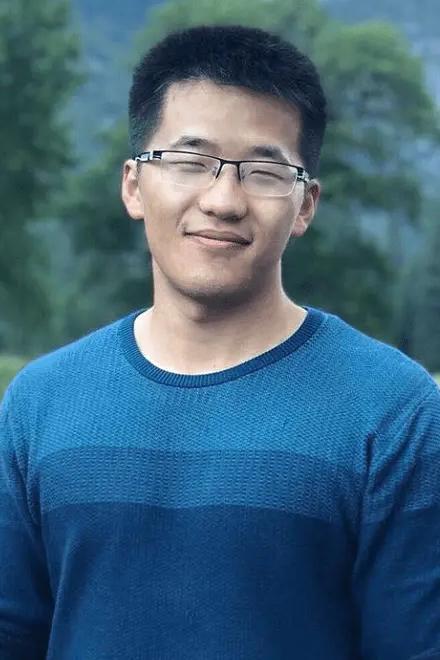
Pan Li
Assistant Professor
School of Electrical and Computer Engineering
Li’s project will improve how scientists understand and use modern graph-structured data, which has become incredibly complex. Graphs are a fundamental tool for modeling networks of data, like interactions among brain neurons or even social relationships among people. But the complexity of modern data has created new challenges in the field, and researchers need robust and trustworthy algorithm tools that can learn from graph-structured data.
Li will work to create models that learn from higher-order relations often missed in traditional graph models, and he will explore deep learning techniques for teasing insights and solutions from graph-structured data. His tools could improve understanding of social and biological networks and improve data processing for high-energy physics research.
Related Content
NSF Awards Sought-After CAREER Funding to 5 Engineering Faculty
BME, ECE, ME early career professors will use the five-year grants to study the brain, advance thermal battery technology, and more.